Building a Data Science Portfolio: Showcasing Your Skills for a Successful Career
By using an excellent portfolio, you can demonstrate to a potential employer that you possess the skills necessary to be successful in a data science position. Learn to develop a strong data science portfolio that might help your employment chances.
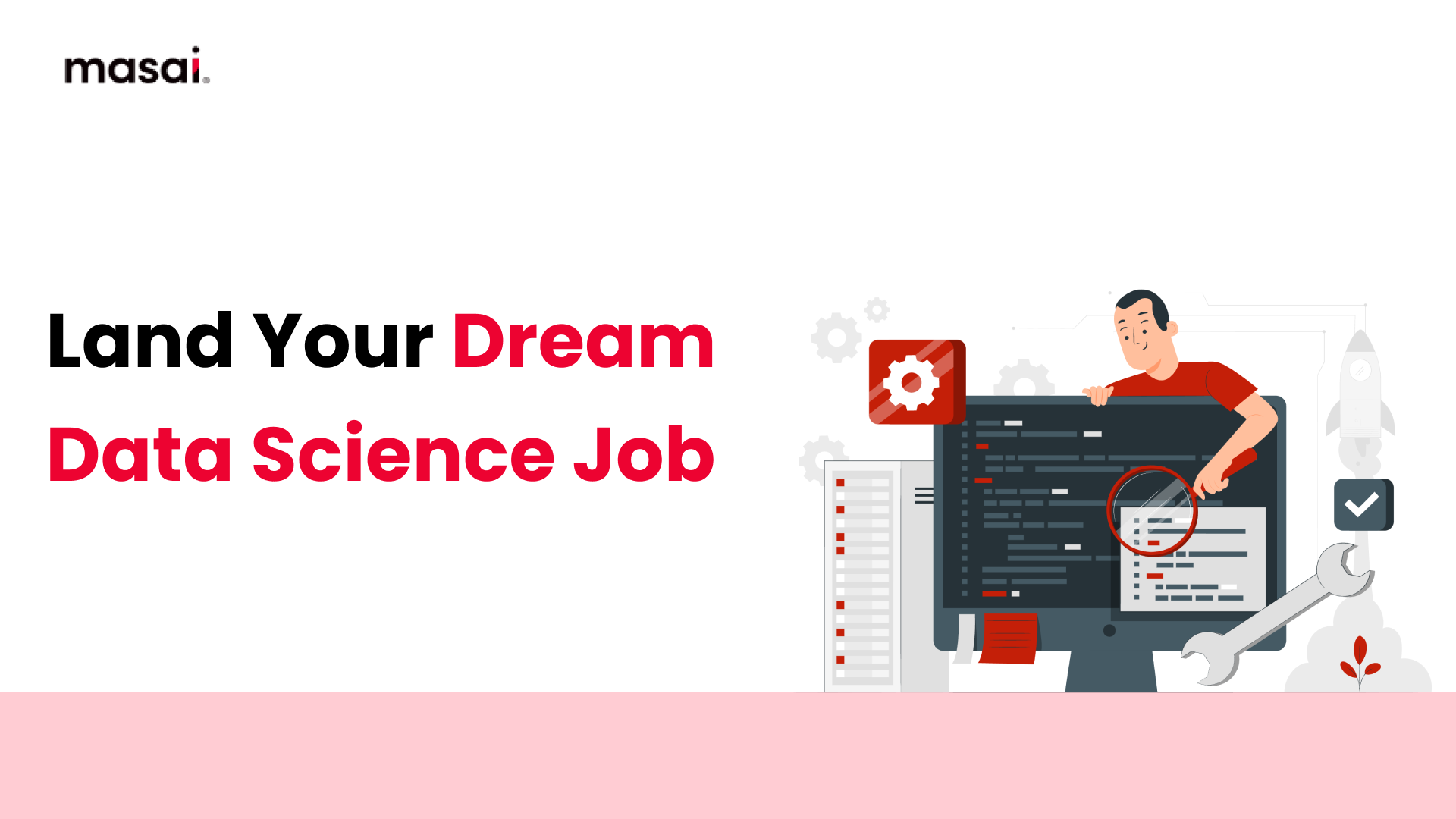
Introduction
Possessing a robust portfolio is not an option in the swiftly changing data science landscape; it is a requirement. A well-organized data science portfolio can demonstrate your technical skills and your ability to think critically and creatively in response to new challenges. This in-depth guide will teach you how to create a data science portfolio highlighting your best work and helping you land your dream job.
How to Put Together a Data Science Portfolio in Easy Steps?
1. Choosing Appropriate Projects
Your portfolio is your story, and each project you present should reflect your desired narrative. Customize your project selection to correspond with the specific role or industry in which you aspire to operate. Your portfolio should reflect your proficiency and enthusiasm in predictive analytics, natural language processing, and data visualization.
2. Demonstrating a Wide Range of Skills
Data science is an interdisciplinary area in which a wide skill set is highly valued. For individuals aspiring to excel in this field, enrolling in top data science courses in India can provide valuable insights and hands-on experience. Include projects demonstrating your programming language knowledge (such as Python or R), machine learning algorithms, data preprocessing, and data visualization. Displaying your adaptability increases your attractiveness to potential employers looking for well-rounded individuals.
3. Contextualization
Your portfolio projects should serve as more than just showcases of your technical skills. Describe the problem you sought to address and the dataset you used. Explain the project's real-world significance and how your approach contributed to a tangible solution. This contextual layering assists viewers in grasping your work's larger narrative.
4. Technical Process Description
It is critical to be transparent while discussing your technological methodology. Dive deeper into the complexities of your projects by explaining the algorithms used, the preprocessing approaches used, and the reasoning behind your decisions. This exhibits not only your breadth of knowledge but also your ability to navigate challenging technical challenges.
5. Seeing the End Results
Data visualization is a vital tool for efficiently communicating findings. Include engaging and instructive data visualizations that communicate the findings of your efforts succinctly. Visual representations, whether a neatly annotated graph or an interactive dashboard, increase the impact of your work.
6. Providing Code Samples
While not all visitors are technically savvy, adding code snippets to your problem-solving journey provides an engaging experience. Code segments that are well-organized and well-commented provide insights into your coding style, best practices, and approach to problem-solving.
7. Recording Problems and Solutions
Authenticity is demonstrated by acknowledging obstacles encountered throughout project execution. Document the difficulties you experienced and the techniques you used to overcome them. This exhibits your adaptability, resilience, and critical thinking abilities.
8. Demonstrating Teamwork and Leadership
Collaboration is common in data science initiatives, and leadership is highly rewarded Highlight instances of group project teamwork or leadership responsibilities. Effective collaboration demonstrates your ability to work smoothly as part of a team, whereas leadership positions demonstrate your ability to coach and inspire others.
9. Ongoing Updates
A static portfolio may not adequately reflect your growth and evolution. Update your portfolio regularly with new projects, skills gained, and milestones reached. This indicates your dedication to continuous learning and progress.
10. User-Friendly and Clean Design
A clean, intuitive design improves the user experience. Throughout your portfolio, use consistent formatting, typography, and color choices. Check that your portfolio looks good on mobile devices.
Conclusion
Building a compelling data science portfolio needs more than merely displaying projects; it necessitates carefully curating experiences, ideas, and talents. Your portfolio captures your technical prowess, problem-solving skills, and growth trajectory. By following the detailed steps outlined in this guide, you will embark on a life-changing journey to create a data science career portfolio that not only stands out from the crowd but also helps you land your dream job in the rapidly evolving field of data science.
FAQs
Should non-technical abilities be included in my portfolio?
Absolutely. Communication, problem-solving, and teamwork are key success factors—showcase times when you effectively presented complicated results or cooperated easily.
Am I allowed to put personal work in my portfolio?
Personal initiatives that demonstrate your initiative and imagination are essential bonuses. However, ensure they are relevant to the area and presented with the same professionalism as professional initiatives.
What role does documentation play in a data science portfolio?
The importance of documentation cannot be overstated. Explicit descriptions of project objectives, techniques, and outcomes demonstrate professionalism and allow a wider audience to understand your work.
Do code samples have to be included in the portfolio?
While not required, code samples offer authenticity and interactivity to your portfolio. They allow prospective companies to evaluate your coding techniques and problem-solving style.